Paper in ECCV 2022 on “Improved Masked Image Generation with Token-Critic”
Abstract
Non-autoregressive generative transformers recently demonstrated impressive image generation performance, and orders of magnitude faster sampling than their autoregressive counterparts. However, optimal parallel sampling from the true joint distribution of visual tokens remains an open challenge. In this paper we introduce Token-Critic, an auxiliary model to guide the sampling of a non-autoregressive generative transformer. Given a masked-and-reconstructed real image, the Token-Critic model is trained to distinguish which visual tokens belong to the original image and which were sampled by the generative transformer. During non-autoregressive iterative sampling, Token-Critic is used to select which tokens to accept and which to reject and resample. Coupled with Token-Critic, a state-of-the-art generative transformer significantly improves its performance, and outperforms recent diffusion models and GANs in terms of the trade-off between generated image quality and diversity, in the challenging class-conditional ImageNet generation.
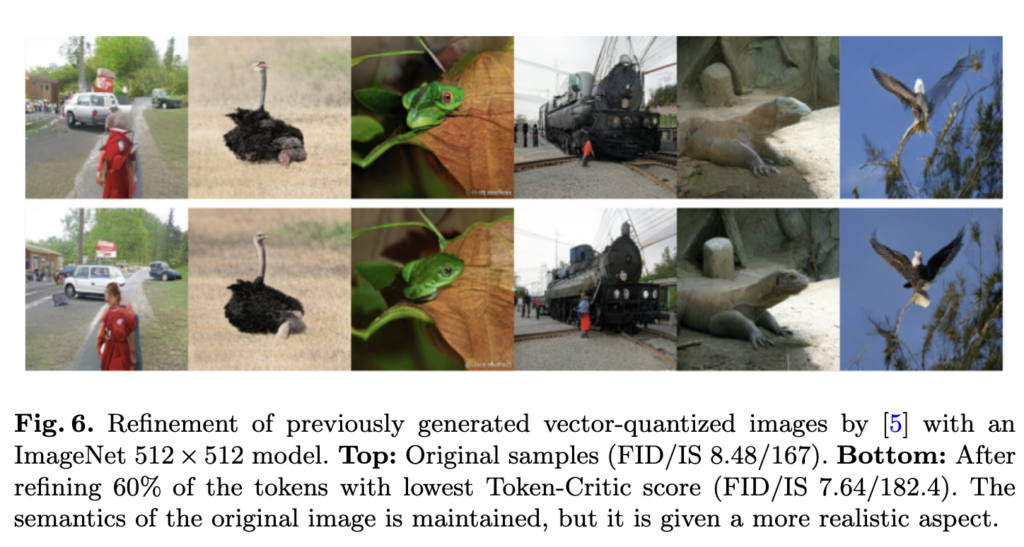
Paper / Citation
Improved Masked Image Generation with Token-Critic Proceedings Article
In: European Conference on Computer Vision (ECCV), arXiv, 2022, ISBN: 978-3-031-20050-2.